3 min read
Powering AI and Automotive Applications with the MIPI Camera Interface
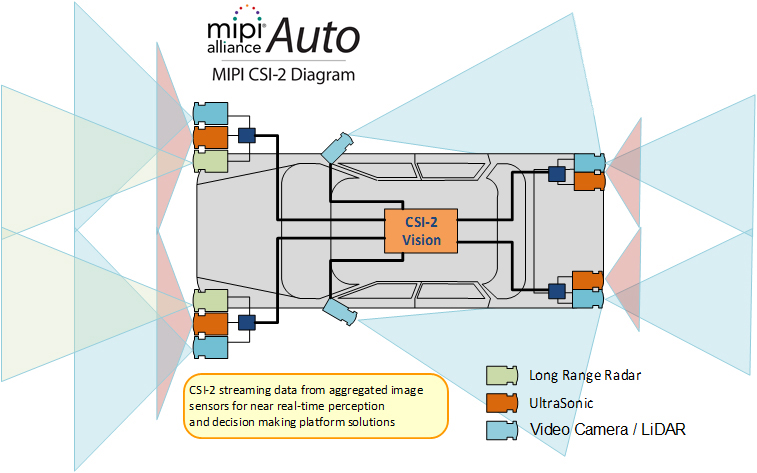
- News & Events
- News
- Blog
Editor’s note: MIPI CSI-2 over C-PHY, D-PHY and the upcoming A-PHY are end-to-end imaging conduit solutions mapped to mobile, client (e.g., notebooks and all-in-one PCs), IoT and autonomous (e.g., automotive and drone) platforms, and support a broad range of imaging and vision applications. This article highlights automotive-related use cases and capabilities using CSI-2 over D-PHY.
The initial version of MIPI CSI-2SM, released in November 2005, was designed to connect cameras to processors in mobile phones. But like many MIPI specifications, MIPI CSI-2 quickly found its way into other applications and has become the de facto standard for camera interfaces for automotive, drones, augmented/virtual reality (AR/VR) headsets and Internet of Things (IoT) devices.
Each new version of MIPI CSI-2 anticipates emerging imaging trends in mobile and mobile-influenced industries, including 1080p, 4K, 8K and beyond video. Since 2009, MIPI CSI-2 has been supported by MIPI D-PHYSM, which is a clock-forwarded synchronous link that provides high noise immunity and high jitter tolerance. Performance is lane-scalable up to 18 Gbps with four-lane (10-wire) MIPI D-PHY v2.1 interface under MIPI CSI-2 v2.1. MIPI D-PHY is ideal for automotive applications such as connecting rear megapixel cameras and high-resolution dashboard displays to the vehicle’s application processor. Figure 1 illustrates how the two MIPI specifications work together.
Figure 1: MIPI CSI-2 and MIPI D-PHY complement each other to provide automotive OEMs and their suppliers with the tools necessary to develop imaging applications.
Enabling AI for Autonomous and Semi-Autonomous Vehicles
Today, drivers are the primary users of rear-view cameras in the sense that they watch their dashboard displays as they are backing up. But AI is another type of user—one that’s rapidly growing because it is capable of identifying obstacles faster than humans. Examples include sensors for radar and LIDAR for Advanced Driver Assistance Systems (ADAS).
Both humans and AI benefit from the increasingly higher resolutions that MIPI CSI-2 supports. Another key advancement is support for greater color depths: up to RAW 20 in MIPI CSI-2 v3.0 (adoption anticipated in spring 2019). The human eye cannot detect the subtle differences that high color depths provide, but AI can.
As a result, MIPI CSI-2 enables the advanced, AI-powered vison capabilities necessary to make autonomous and semi-autonomous vehicles safe and practical. For example, RAW 20 ensures highly nuanced image capture even when the lighting environment changes suddenly and dramatically, such as when a vehicle exits a dimly lit tunnel into bright sunlight. This example also highlights how MIPI CSI-2 supports image sensors around the entire vehicle, including at the front for detecting pedestrians and other vehicles, and on the sides for alerting drivers when they’re drifting out of their lanes.
High color depth is among the many ways that MIPI CSI-2 enables a wide variety of ADAS technologies—including when AI is the driver. Other examples include:
- Support for up to 32 virtual channels (over C-PHY); up to 16 virtual channels over D-PHY. This ensures that MIPI CSI-2 can accommodate the large number of image sensors found in today’s vehicles. The more channels there are, the more sources of data that AI has to understand the rapidly changing environment around a vehicle (Figure 2). Even in relatively static environments, AI still needs multiple image sensors to make more accurate decisions. One example is self-parking, a maneuver that requires up to six cameras in today’s vehicles.
Figure 2: Support for up to 32 virtual channels (over C-PHY) gives a vehicle’s AI more input for making decisions.
- Latency Reduction and Transport Efficiency (LRTE). As the number of sensors grows, LRTE streamlines their aggregation and minimizes transport latency (Figure 3). This ensures that AI gets the data it needs quickly so it can respond to changing conditions faster.
Figure 3: LRTE reduces frame transport latency to facilitate real-time perception and decision-making applications.
- (Differential Pulse Code Modulation) DPCM 12-10-12 compression. This new compression scheme reduces bandwidth while delivering superior SNR images, removing more compression artifacts than the scheme in MIPI CSI-2 v1.3.
- Galois Field Scrambling. This feature reduces power spectral density (PSD) emissions and minimizes radio interference, keeping the system immune to sensitive components nearby.
These features and capabilities are among the ways that MIPI CSI-2 and MIPI D-PHY enable deep learning, a subset of machine learning that will enable even more sophisticated vehicular AI applications. Deep learning mimics the human brain, so it requires an enormous amount of memory and bandwidth, as well as real-time data connectivity between sensors and processors. Figure 4 summarizes many of deep learning’s unique requirements, which MIPI Alliance is continually working to support through its roadmap.
Figure 4: Deep learning enables next-generation vehicular AI applications.
To learn more about how MIPI CSI-2 and MIPI D-PHY are enabling safer, better vehicles today and tomorrow, listen to the MIPI DevCon Seoul presentation “Powering AI and Automotive Applications with the MIPI Camera Interface” and download the accompanying slide deck.
Note: MIPI A-PHY, a longer reach physical layer specification (up to 15m) targeted for ADS, ADAS and other surround sensor applications, is currently under development. Visit the MIPI website to learn more about this new specification.